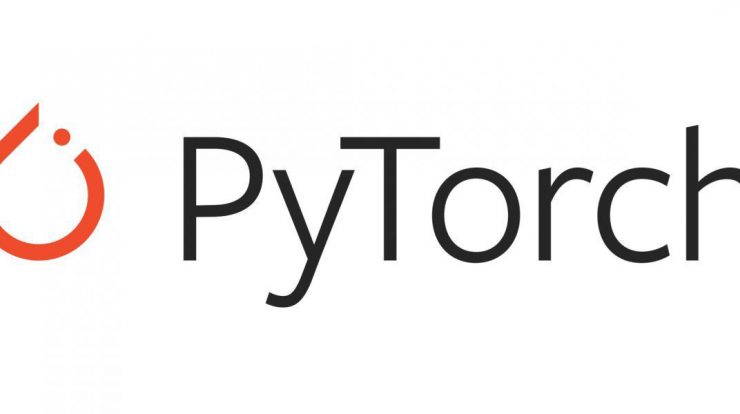
Description About PyTorch
PyTorch is an optimized tensor library primarily used for Deep Learning applications using GPUs and CPUs. It is an open-source machine learning library for Python, mainly developed by the Facebook AI Research team. It is one of the widely used Machine learning libraries, others being TensorFlow and Keras.
Moving along to questions and answers
1. What is PyTorch?
Answer: PyTorch is a part of computer software based on torch library, which is an open-source Machine learning library for Python. It is a deep learning framework that was developed by the Facebook artificial intelligence research group. It is used for applications such as Natural Language Processing and Computer Vision.
2. Why do we use activation function in Neural Network
Answer: To determine the output of the neural network, we use the Activation Function. Its main task is to do mapping of resulting values in between 0 to 1 or -1 to 1 etc. The activation functions are basically divided into two types:
- Linear Activation Function
- Non-linear Activation Function
3. Why use PyTorch for Deep learning?
Answer: In Deep learning tools, PyTorch plays an important role, and it is a subset of machine learning, and its algorithm works on the human brain. There is the following reason for which we prefer PyTorch:
- PyTorch allows us to define our graph dynamically.
- PyTorch is great for deep-earning research and provides maximum flexibility and speed.
4. What are the essential elements of PyTorch?
Answer: There are the following elements that are essential in PyTorch:
- PyTorch tensors
- PyTorch NumPy
- Mathematical operations
- Autographed Module
- Optim Module
- nn Module
5. Why do we prefer the sigmoid activation function rather than other functions?
Answer: The Sigmoid Function curve looks like S-shape and the reason why we prefer a sigmoid rather than other is a sigmoid function exists between 0 to 1. This is especially used for the models where we have to predict the probability as an output.
6. What is the attributes of Tensor?
Answer: Each torch.Tensor has a torch.device, torch.layout, and torch.dtype. The torch.dtype defines the data type, torch.device represents the device on which a torch.Tensor is allocated, and torch.layout represents the memory layout of a torch.Tensor.
7. What are Tensors?
Answer: Tensors play an important role in deep learning with PyTorch. In simple words, we can say, this framework is completely based on tensors. A tensor is treated as a generalized matrix. It could be a 1D tensor (vector), 2D tensor(matrix), 3D tensor(cube) or 4D tensor(cube vector).
8. What do you mean by Feed-Forward?
Answer: “Feed-Forward” is a process through which we receive an input to produce some kind of output to make some kind of prediction. It is the core of many other important neural networks such as convolution neural networks and deep neural network./p>
In the feed-forward neural network, there are no feedback loops or connections in the network. Here is simply an input layer, a hidden layer, and an output layer.
9. What is the difference between Anaconda and Miniconda?
Answer: Anaconda is a set of hundreds of packages including conda, numpy, scipy, ipython notebook, and so on. Miniconda is a smaller alternative to anaconda.
10. What are the levels of abstraction?
Answer: There are three levels of abstraction, which are as follows:
- Tensor: Tensor is an imperative n-dimensional array that runs on GPU.
- Variable: It is a node in the computational graph. This stores data and gradient.
- Module: Neural network layer will store the state of the otherwise learnable weight.
11. What is the difference between Conv1d, Conv2d, and Conv3d?
Answer: There is no big difference between the three of them. The Conv1d and Conv2D is used to apply 1D and 2D convolution. The Conv3D is used to apply 3D convolution over an input signal composed of several input planes.
12. How do we check GPU usage?
Answer: There are the following steps to check GPU usage:
- Use the window key + R to open the run command.
- Type the dxdiag.exe command and press enter to open DirectXDiagnostic Tool.
- Click on the Display tab.
- Under the drivers, on the right side, check the Driver model information.
13. Are tensor and matrix the same?
Answer: We can’t say that tensor and matrix are the same. Tensor has some properties through which we can say both have some similarities such as we can perform all the mathematical operations of the matrix in tensor.
A tensor is a mathematical entity that lives in a structure and interacts with other mathematical entities. If we transform the other entities in the structure in a regular way, then the tensor will obey a related transformation rule. This dynamical property of the tensor makes it different from the matrix.
14. What do you understand from the word Backpropagation?
Answer: “Backpropagation” is a set of algorithms that are used to calculate the gradient of the error function. This algorithm can be written as a function of the neural network. These algorithms are a set of methods that are used to efficiently train artificial neural networks following a gradient descent approach that exploits the chain rule.
15. What is the MNIST dataset?
Answer: The MNIST dataset is used in Image Recognition. It is a database of various handwritten digits. The MNIST dataset has a large amount of data which is commonly used to demonstrate the true power of deep neural networks.
16. What is the use of a torch.from_numpy()?
Answer: The torch.from_numpy() is one of the important properties of the torch which places an important role in tensor programming. It is used to create a tensor from numpy.ndarray. The ndarray and return tensor share the same memory. If we do any changes in the returned tensor, then it will reflect the ndaaray also.
17. What is Convolutional Neural Network?
Answer: Convolutional Neural Network is the category to do image classification and image recognition in neural networks. Face recognition, scene labeling, objects detections, etc., are the areas where convolutional neural networks are widely used. The CNN takes an image as input, which is classified and processed under a certain category such as dog, cat, lion, tiger, etc.
18. What is the CIFAR-10 dataset?
Answer: It is a collection of the color image which is commonly used to train machine learning and computer vision algorithms. The CIFAR 10 dataset contains 50000 training images and 10000 validation images such that the images can be classified between 10 different classes.
19. What is variable and autograd.variable?
Answer: A variable is a package that is used to wrap a tensor. The autograd.variable is the central class for the package. The torch.autograd provides classes and functions for implementing automatic differentiation of arbitrary scalar-valued functions. It needs minimal changes to the existing code. We only need to declare tensor for which gradients should be computed with the requires_grad=True keyword.
20. What is the difference between DNN and CNN?
Answer: The deep neural network is a kind of neural network with many layers. “Deep” means that the neural network has a lot of layers that look like deep stuck of layers in the network. The convolutional neural network is another kind of deep neural network. The Convolutional Neural Network has a convolution layer, which is used filters to convolve an area in input data to a smaller area, detecting important or specific parts within the area. The convolution can be used on the image as well as text.
21. What is the difference between the CIFAR-10 and CIFAR-100 dataset?
Answer: The CIFAR 10 dataset contains 50000 training images and 10000 validation images such that the images can be classified between 10 different classes. On the other hand, CIFAR-100 has 100 classes, which contain 600 images per class. There are 100 testing images and 500 training images per class.
22. How do we find the derivatives of the function in PyTorch?
Answer: The derivatives of the function are calculated with the help of the Gradient. There are four simple steps through which we can calculate derivative easily.
These steps are as follows:
- Initialization of the function for which we will calculate the derivatives.
- Set the value of the variable which is used in the function.
- Compute the derivative of the function by using the backward () method.
- Print the value of the derivative using grad.
23. What are the advantages of PyTorch?
Answer: There are the following advantages of Pytorch:
- PyTorch is very easy to debug.
- It is a dynamic approach for graph computation.
- It is a very fast deep learning training than TensorFlow.
- It increased developer productivity.
- It is very easy to learn and simpler to code.
24. What do you mean by convolution layer?
Answer: The convolution layer is the first layer in Convolutional Neural Network. It is the layer to extract the features from an input image. It is a mathematical operation that takes two inputs such as an image matrix and a kernel or filter.
25. What do you mean by Linear Regression?
Answer: Linear Regression is a technique or way to find the linear relation between the dependent variable and the independent variable by minimizing the distance. It is a supervised machine learning approach that is used for the classification of order discrete categories.
26. What is the difference between PyTorch and TensorFlow?
Answer:
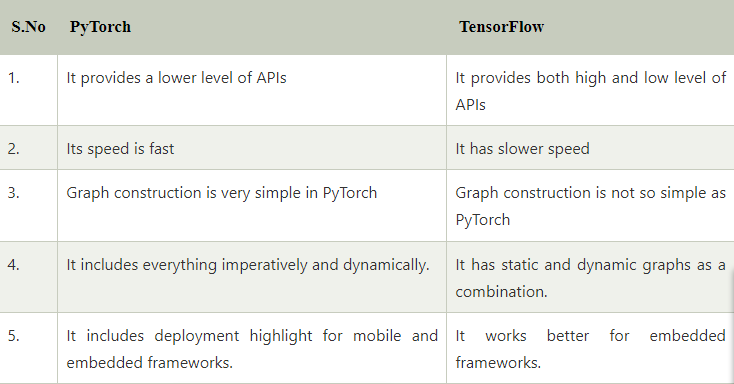
27. What do you mean by Stride?
Answer: Stride is the number of pixels that are shifted over the input matrix. We move the filters to 1 pixel at a time when the stride is equaled to 1.
28. What is Loss Function?
Answer: The loss function is bread and butter for machine learning. It is quite simple to understand and used to evaluate how well our algorithm models our dataset. If our prediction is completely off, then the function will output a higher number else it will output a lower number.
29. Give anyone the difference between batch, stochastic, and mini-batch gradient descent?
Answer:
- Stochastic Gradient Descent: In SGD, we use only a single training example for the calculation of gradient and parameters.
- Batch Gradient Descent: In BGD, we calculate the gradient for the whole dataset and perform the updation at each iteration.
- Mini-batch Gradient Descent: Mini-batch Gradient Descent is a variant of Stochastic Gradient Descent. In this gradient descent, we used mini-batch of samples instead of a single training example.
30. What do you mean by Padding?
Answer: “Padding is an additional layer which can add to the border of an image.” It is used to overcome the
- Shrinking outputs
- Losing information on the corner of the image.
31. What is the use of MSELoss, CTCLoss, and BCELoss function?
Answer: MSE stands for Mean Squared Error, which is used to create a criterion that measures the mean squared error between each element in an input x and target y. The CTCLoss stands for Connectionist Temporal Classification Loss, which is used to calculate the loss between continuous time series and target sequence. The BCELoss(Binary Cross Entropy) is used to create a criterion to measures the Binary Cross Entropy between the target and the output.
32. What is an auto-encoder?
Answer: It is a self-government machine learning algorithm that uses the backpropagation principle, where the target values are equal to the inputs provided. Internally, it has a hidden layer that manages a code used to represent the input.
33. What is the pooling layer.
Answer: The pooling layer plays a crucial role in pre-processing of an image. The pooling layer reduces the number of parameters when the images are too large. Pooling is “downscaling” of the image which is obtained from the previous layers
34. Give anyone the difference between torch.nn and torch.nn.functional?
Answer: The torch.nn provides us with many more classes and modules to implement and train the neural network. The torch.nn.functional contains some useful functions like activation function and convolution operation, which we can use. However, these are not full layers, so if we want to define a layer of any kind, we have to use torch.nn.
35. What is the Autograd module in PyTorch?
Answer: The Autograd module is an automatic differentiation technique that is used in PyTorch. It is more powerful when we are building a neural network. There is a recorder that records each operation that we have performed, and then it replays it back to compute our gradient.
36. What is Max Pooling?
Answer: Max pooling is a sample-based discrete process whose main objective is to reduce its dimensionality, downscale an input representation. And allow for the assumption to be made about features contained in the sub-region binned.
37. What do you mean by Mean Squared Error?
Answer: The mean squared error tells us about how close a regression line to a set of points. Mean squared error does this by taking the distance from the points to the regression line and squaring them. Squaring is required to remove any negative signs.
38. What is the optim module in PyTorch?
Answer:

39. What is Average Pooling?
Answer: Down-scaling will perform through average pooling by dividing the input into rectangular pooling regions and will compute the average values of each region.
40. What is perceptron?
Answer: Perceptron is a single-layer neural network, or we can say a neural network is a multi-layer perceptron. Perceptron is a binary classifier, and it is used in supervised learning. A simple model of a biological neuron in an artificial neural network is known as Perceptron.
41. What is nn module in PyTorch?
Answer: PyTorch provides the torch.nn module to help us in creating and training of the neural network. We will first train the basic neural network on the MNIST dataset without using any features from these models. The torch.nn provides us with many more classes and modules to implement and train the neural network.
42. What is Sum Pooling?
Answer: The sub-region for sum pooling or mean pooling will set the same as for max-pooling but instead of using the max function we use sum or mean.
43. What is the Activation function?
Answer: A neuron should be activated or not, is determined by an activation function. The Activation function calculates a weighted sum and further adds bias with it to give the result. The Neural Network is based on the Perceptron, so if we want to know the working of the neural network, then we have to learn how perception works.
44. What is the command to install PyTorch in windows using Conda and pip?
Answer:

45. What do you mean by a fully connected layer?
Answer: The fully connected layer is a layer in which the input from the other layers will be flattened into a vector and sent. It will transform the output into the desired number of classes by the network.
46. How Neural Network differs from Deep Neural Network?
Answer: Neural Network and Deep Neural Network both are similar and do the same thing. The difference between NN and DNN is that there can be only one hidden layer in the neural network, but in a deep neural network, there is more than one hidden layer. Hidden layers play an important role to make an accurate prediction.
47. What is torch.cuda?
Answer: The torch.cuda is a package that adds support for CUDA tensor type. CUDA tensor type implements the same function as CPU tensor but utilizes the GPU for computation.
48. What is the Softmax activation function?
Answer: The Softmax function is a wonderful activation function that turns numbers aka logits into probabilities that sum to one. Softmax function outputs a vector that represents the probability distributions of a list of potential outcomes.
49. Why it is difficult for the network is showing the problem?
Answer: Ann works with numerical information, and the problems are translated into numeric values before being introduced to ANN. This is the reason by which it is difficult to show the problem to the network.
50. What is the difference between Type1 and Type2 errors?
Answer: Type 1 error is the false positive value, and Type 2 error is a false negative value. Type I error represents when something is happening. Type II errors are describing that there nothing is wrong where something is not right.
- How to Choose Wireless Access Points for Office - December 13, 2024
- Online Real Estate Courses: Navigating the Shift to Digital Education - December 13, 2024
- From Concept to Implementation: IoT Services Redefining Modern Solutions - December 13, 2024