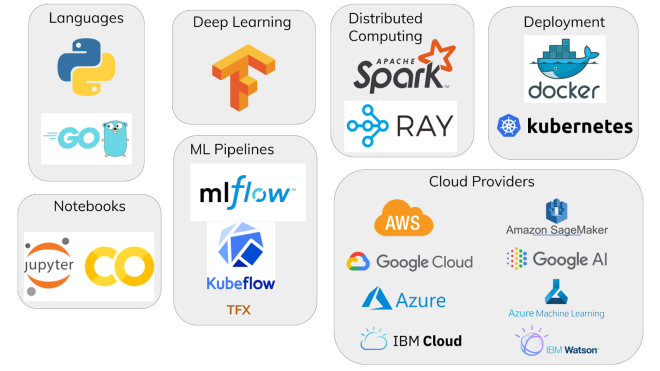
As the realm of data continues to expand, the ethical implications and responsible use of data become increasingly crucial. Here’s an overview of some notable Data Ethics Tools in 2024:
Assessment and Auditing Tools:
- IBM AI Fairness 360: An open-source toolkit that helps identify and mitigate potential bias in machine learning models, offering various fairness metrics and algorithms.
- Google AI Platform Explainable AI: A suite of tools within Google’s cloud platform that provides insights into model behavior and helps explain predictions, promoting transparency and trust.
- Algorithmic Justice League’s Checklist for Ethical AI: A community-developed framework offering guiding principles and questions to assess the fairness, accountability, and transparency of AI systems.
Impact Assessment and Mitigation Tools:
- World Economic Forum’s Global AI Assessment: A comprehensive framework and platform for evaluating the potential impacts of AI across various domains, helping identify and address risks and opportunities.
- Montreal Declaration for Responsible AI: A set of principles and recommendations for the development and use of trustworthy and ethical AI, promoting responsible research and development practices.
- Responsible AI Institute (RAI) Tools and Resources: A collection of tools, frameworks, and resources developed by RAI to support responsible AI development and deployment, including bias detection and mitigation techniques.
Data Privacy and Security Tools:
- Differential privacy libraries: Open-source libraries like OpenDP and TensorFlow Privacy provide methods for adding noise to data while preserving its utility, protecting individual privacy during analysis.
- Federated learning frameworks: Tools like TensorFlow Federated and PySyft enable collaborative machine learning models without sharing raw data, protecting sensitive information and privacy.
- Data encryption and anonymization tools: Various tools and techniques like homomorphic encryption and anonymization algorithms help secure and protect data while enabling its use for analysis.
Data ethics is an ongoing process, not a single solution. These tools are valuable resources, but ethical considerations require careful evaluation, human judgment, and ongoing monitoring throughout the data lifecycle.
Additional Factors to Consider:
- Industry and regulatory landscape: Understand relevant regulations and ethical guidelines in your specific domain.
- Organizational values and context: Align data practices with your organization’s ethical principles and mission.
- Stakeholder engagement: Involve diverse stakeholders in discussions and decision-making about data use.
Say goodbye to the hassles of bike ownership! MotoShare.in offers affordable rentals, whether you need a scooter for errands, a bike for a road trip, or a reliable ride to explore new cities.