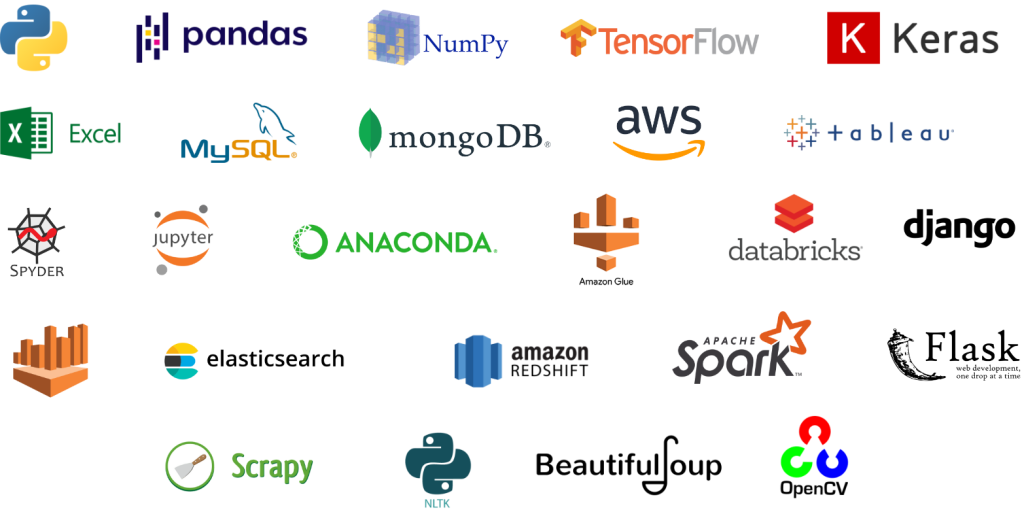
As data continues to reign supreme in various fields, effective data science platforms are more crucial than ever in 2024. Here are some of the top contenders to consider, along with their key strengths:
End-to-End Solutions:
- Dataiku: This platform stands out for its user-friendly interface and drag-and-drop capabilities, catering to a diverse range of users, from data analysts to data scientists. It streamlines the entire data science workflow, from data preparation and exploration to model building and deployment.
- KNIME: Another user-friendly option, KNIME shines with its modular design and visual workflows. It allows for easy collaboration and customization, making it suitable for data science teams of all sizes.
- RapidMiner: Boasting a wide range of features and pre-built components, RapidMiner empowers users to build, train, and deploy machine learning models efficiently. Its visual interface and automation capabilities simplify complex tasks.
Open-Source Powerhouses:
- Jupyter Notebook: This versatile open-source tool has become a mainstay for data exploration, analysis, and visualization. Its interactive nature and support for various programming languages make it a popular choice for data scientists and researchers.
- RStudio: Widely used for statistical analysis and data visualization, RStudio offers a powerful and customizable environment for data scientists of all levels. Its open-source nature and vast community contribute to its popularity.
- Scikit-learn: This library built on top of Python provides a comprehensive set of tools for machine learning tasks, including classification, regression, and clustering. Its simplicity and ease of use make it a favorite among data science practitioners.
Cloud-Based Platforms:
- Amazon SageMaker: This managed service by AWS provides a comprehensive suite of tools and infrastructure for building, training, and deploying machine learning models in the cloud. Its scalability and integration with other AWS services make it an attractive option for businesses already invested in the AWS ecosystem.
- Microsoft Azure Machine Learning: Azure’s cloud-based platform offers various tools and services for developing and deploying machine learning models, including deep learning. It integrates well with other Azure services and caters to diverse data science needs.
- Google Cloud AI Platform: Google’s cloud platform provides pre-trained models, tools, and infrastructure for building and deploying deep learning models. Its integration with other Google Cloud services and focus on AI capabilities make it a strong contender.
Choosing the Right Platform:
The best data science platform for you depends on your specific needs and budget. Consider factors such as:
- Team size and expertise: Are you working as an individual or in a team? What are your team members’ skillsets?
- Project complexity: Are you tackling a simple data analysis task or a complex machine learning project?
- Open-source vs. commercial: Do you prefer the flexibility and cost-effectiveness of open-source tools or the convenience and support of commercial platforms?
- Cloud vs. on-premise: Do you need a cloud-based solution or on-premises infrastructure?
- Budget: How much are you willing to spend on a data science platform?
Say goodbye to the hassles of bike ownership! MotoShare.in offers affordable rentals, whether you need a scooter for errands, a bike for a road trip, or a reliable ride to explore new cities.